These are my sketchnotes for Sam Charrington’s podcast This Week in Machine Learning and AI about Neuroevolution: Evolving Novel Neural Network Architectures with Kenneth Stanley:
Sketchnotes from TWiMLAI talk #94: Neuroevolution: Evolving Novel Neural Network Architectures with Kenneth Stanley
You can listen to the podcast here.
Kenneth studied under TWiML Talk #47 guest Risto Miikkulainen at UT Austin, and joined Uber AI Labs after Geometric Intelligence , the company he co-founded with Gary Marcus and others, was acquired in late 2016.
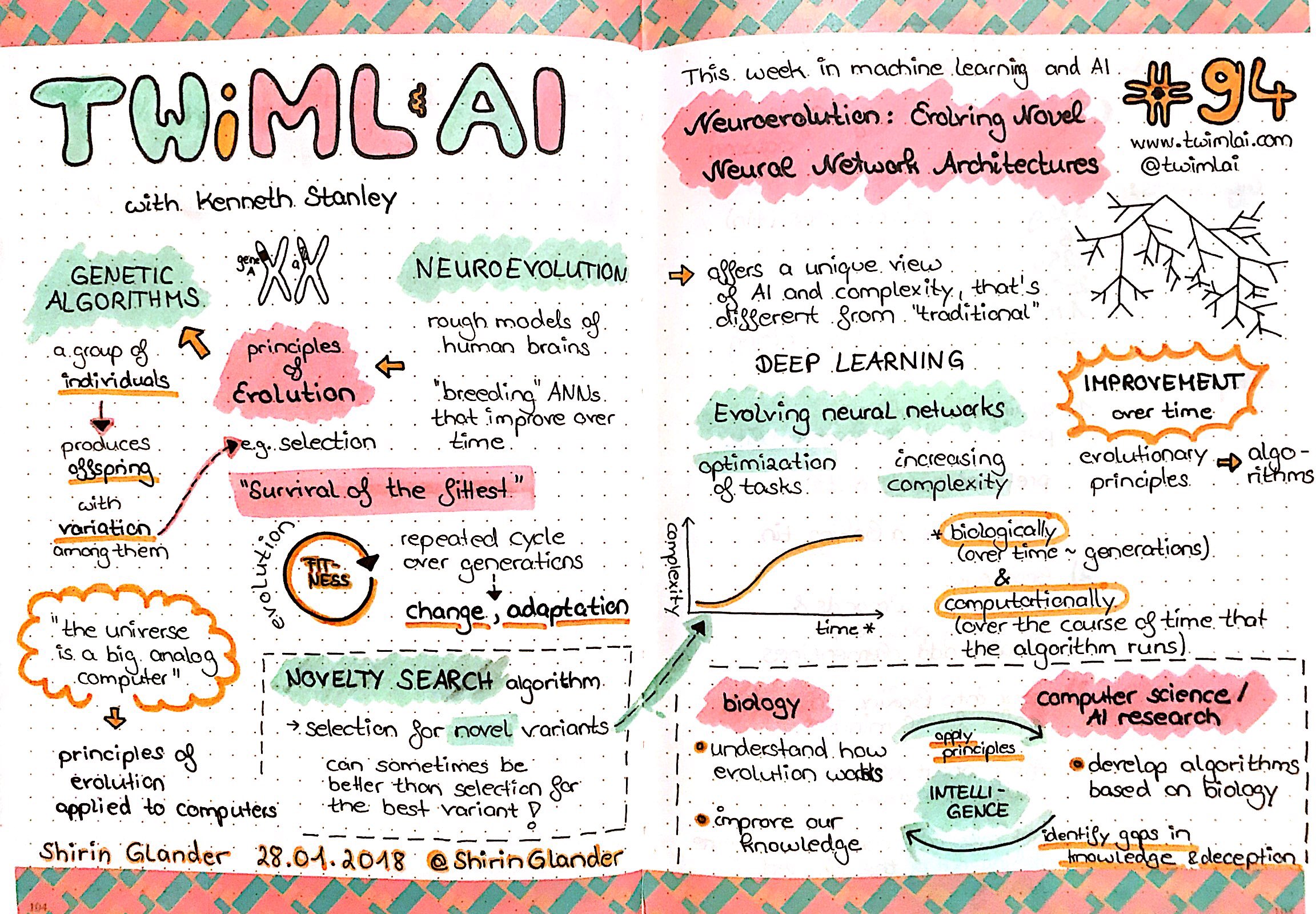