Registration is now open for my 1.5-day workshop on how to develop end-2-end from a Keras/TensorFlow model to production.
It will take place on February 21st & 22nd in Berlin, Germany. The workshop will cost 950.00 Euro + MwST. We will start at 9 am on Thursday and finish around 3 pm on Friday.
Please register by sending an email to shirin.glander@gmail.com with the following information:
name company/institute/affiliation address for invoice phone number reference to this blog The course material will be in English and we will speak a mix of German and English, depending on the participants’ preferences.
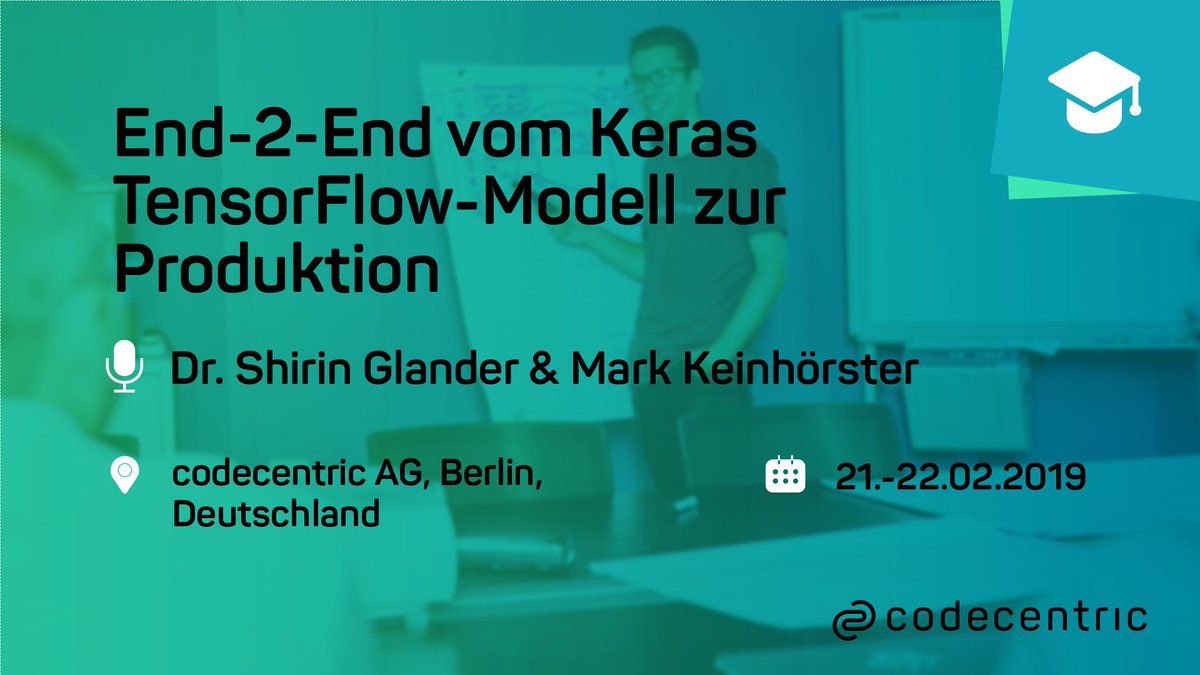